Hyperparameter Optimization, Neural Architecture Search, and Algorithm Selection: The Keys to Unlocking Machine Learning Excellence

In the rapidly evolving landscape of machine learning, hyperparameter optimization, neural architecture search, and algorithm selection have emerged as indispensable tools for unlocking the full potential of models. These techniques empower practitioners to meticulously tune models, craft optimal architectures, and select the most appropriate algorithms, resulting in significant performance enhancements, heightened efficiency, and unmatched accuracy. 4.3 out of 5 This comprehensive guide delves into the intricate details of these transformative concepts, providing a thorough understanding of their workings, benefits, and practical applications. By harnessing the power of these techniques, you can elevate your machine learning models to new heights, achieving remarkable outcomes that were once thought unattainable. Hyperparameter optimization encompasses a set of techniques aimed at finding the optimal values for the hyperparameters of a machine learning model. Unlike model parameters, which are learned during the training process, hyperparameters govern the learning process itself. They control aspects such as the learning rate, batch size, and regularization strength. Optimizing hyperparameters is crucial because they exert a profound influence on model performance. By identifying the optimal settings, you can dramatically enhance accuracy, reduce overfitting, and accelerate training time. Various methods exist for performing hyperparameter optimization. Grid search, a straightforward approach, involves evaluating the model's performance across a predefined grid of hyperparameter values. Random search, on the other hand, randomly samples hyperparameter configurations, offering a more efficient alternative for large search spaces. Bayesian optimization leverages probabilistic models to guide the search process, iteratively updating the probability distribution of promising hyperparameter combinations. More sophisticated techniques, such as evolutionary algorithms and reinforcement learning, have also shown promise in hyperparameter optimization. Neural architecture search (NAS) is a cutting-edge technique that empowers you to automate the design of neural network architectures. Traditionally, designing network architectures was a manual and time-consuming process, often relying on trial and error. NAS algorithms leverage machine learning to search through a vast space of possible architectures, efficiently identifying those that are most suitable for the given task. This process involves training a meta-model that evaluates the performance of different architectures. NAS algorithms can be broadly classified into two categories: gradient-based and reinforcement learning-based. Gradient-based methods, such as differentiable architecture search (DARTS),utilize gradients to guide the search process. Reinforcement learning-based methods, on the other hand, train a reinforcement learning agent to navigate the architecture search space. Recent advancements in NAS have witnessed the emergence of evolutionary algorithms, which leverage principles of natural selection to evolve optimal architectures. Collaborative NAS methods, where multiple agents cooperate to search the architecture space, have also shown promising results. Algorithm selection involves choosing the most appropriate machine learning algorithm for the given task. With a plethora of algorithms available, selecting the optimal one can be a daunting task. Algorithm selection methods aim to analyze the characteristics of the dataset and task, identifying the algorithm that is likely to perform best. This analysis considers factors such as the data type, problem complexity, and computational resources available. Several methods can be employed for algorithm selection. Empirical evaluation, a straightforward approach, involves training and comparing different algorithms on a representative dataset. Statistical methods, such as meta-learning, leverage past experiences to predict the performance of different algorithms on new tasks. Model-based methods construct a model to estimate the performance of different algorithms. More recently, automated algorithm selection frameworks have gained popularity, combining multiple methods to provide comprehensive recommendations. Hyperparameter optimization, neural architecture search, and algorithm selection are indispensable tools in the modern machine learning landscape. By mastering these techniques, you can unlock the full potential of your models, achieving unprecedented levels of performance, efficiency, and accuracy. Whether you are a seasoned practitioner or just starting your journey in machine learning, this guide has provided you with a comprehensive understanding of these transformative concepts. Embrace these techniques to empower your models and drive innovation in your projects. The future of machine learning holds countless possibilities, and these techniques will continue to play a pivotal role in shaping the landscape. Stay abreast of the latest advancements and continue to explore the boundless potential of machine learning.Language : English File size : 66179 KB Text-to-Speech : Enabled Screen Reader : Supported Enhanced typesetting : Enabled Print length : 312 pages Paperback : 383 pages Item Weight : 1.26 pounds Dimensions : 5.5 x 0.96 x 8.5 inches Hyperparameter Optimization: Fine-tuning Model Performance
What is Hyperparameter Optimization?
Methods of Hyperparameter Optimization
Benefits of Hyperparameter Optimization
Neural Architecture Search: Crafting Optimal Model Architectures
What is Neural Architecture Search?
Methods of Neural Architecture Search
Benefits of Neural Architecture Search
Algorithm Selection: Choosing the Right Tool for the Job
What is Algorithm Selection?
Methods of Algorithm Selection
Benefits of Algorithm Selection
4.3 out of 5
Language | : | English |
File size | : | 66179 KB |
Text-to-Speech | : | Enabled |
Screen Reader | : | Supported |
Enhanced typesetting | : | Enabled |
Print length | : | 312 pages |
Paperback | : | 383 pages |
Item Weight | : | 1.26 pounds |
Dimensions | : | 5.5 x 0.96 x 8.5 inches |
Do you want to contribute by writing guest posts on this blog?
Please contact us and send us a resume of previous articles that you have written.
Book
Novel
Page
Chapter
Text
Story
Genre
Reader
Library
Paperback
E-book
Magazine
Newspaper
Paragraph
Sentence
Bookmark
Shelf
Glossary
Bibliography
Foreword
Preface
Synopsis
Annotation
Footnote
Manuscript
Scroll
Codex
Tome
Bestseller
Classics
Library card
Narrative
Biography
Autobiography
Memoir
Reference
Encyclopedia
Addie Joss
I P Mayers
Tina Mischke
Eric Simons
Adele J Jean
Adrian Streather
Ahmad Taher Azar
Alan J Izenman
Delphi Classics
George Constable
Alexander Hartung
Alain Abraham
Alan Allport
J C Sum
Susan Jaques
Julie Buxbaum
Akmal Izzat
Ai Publishing
Julia M Ritter
Rev Johannes Myors
Light bulbAdvertise smarter! Our strategic ad space ensures maximum exposure. Reserve your spot today!
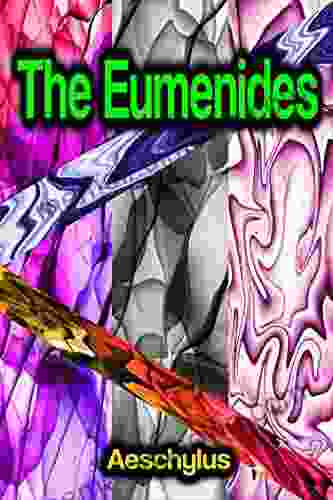

- Quentin PowellFollow ·16.6k
- Douglas FosterFollow ·3k
- Danny SimmonsFollow ·8k
- Yukio MishimaFollow ·3.6k
- Fernando BellFollow ·6.2k
- Billy FosterFollow ·10.6k
- Thomas HardyFollow ·12.8k
- John ParkerFollow ·12.2k


Memories of Disneyland Maintenance: Unlocking the Hidden...
A Nostalgic Journey...


Inspire Young Minds: Unlocking the Wisdom of Chanakya in...
A Journey of...
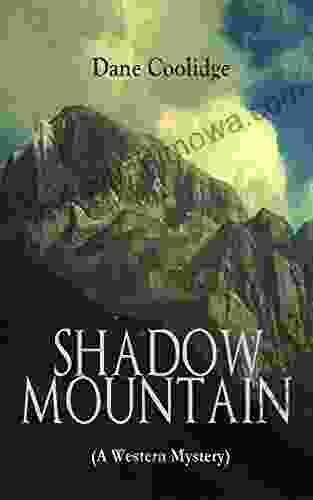

Unravel the Enigmatic Mystery of Shadow Mountain in this...
Prologue: A...
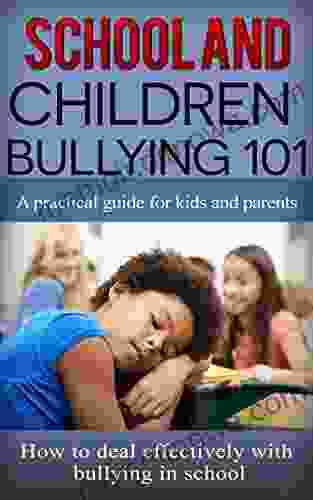

School and Children Bullying: A Beginner's Guide for Kids...
Bullying is a serious problem that affects...


The Crematory: 31 Horrifying Tales from the Dead
Prepare yourself for a spine-chilling...
4.3 out of 5
Language | : | English |
File size | : | 66179 KB |
Text-to-Speech | : | Enabled |
Screen Reader | : | Supported |
Enhanced typesetting | : | Enabled |
Print length | : | 312 pages |
Paperback | : | 383 pages |
Item Weight | : | 1.26 pounds |
Dimensions | : | 5.5 x 0.96 x 8.5 inches |